Learning like humans, machines extend the reach of research
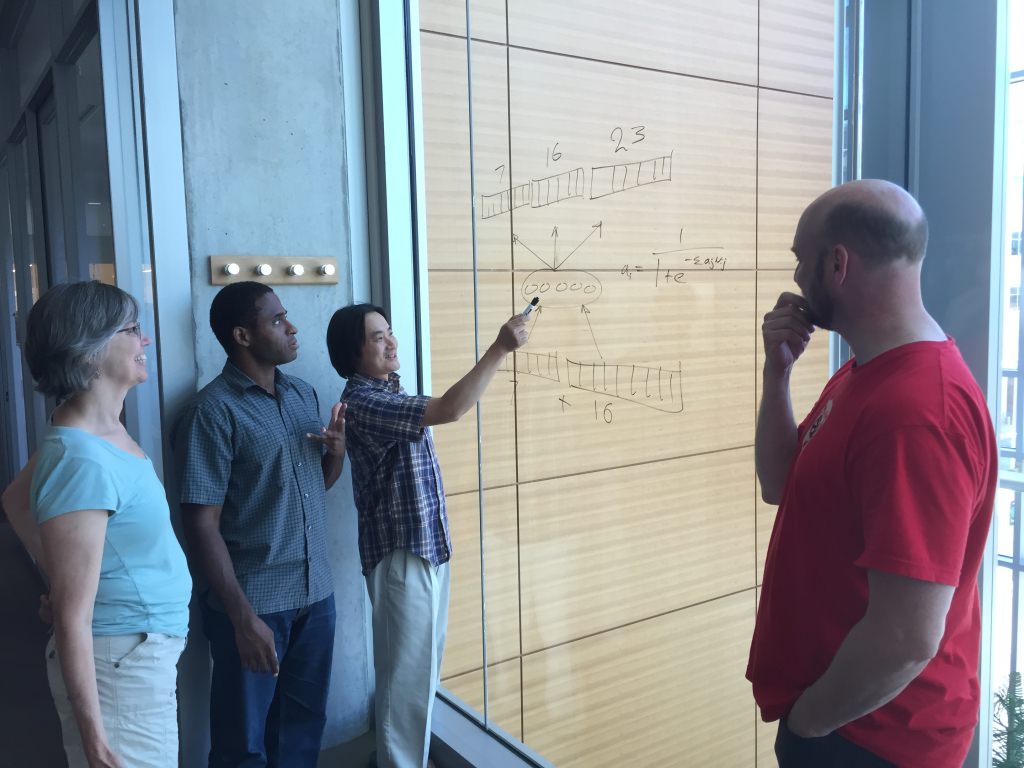
Tim Rogers (right) is principal investigator of LUCID (Learning, Understanding, Cognition, Intelligence and Data Science). LUCID was created to train graduate students to work on problems with the help of machine learning, in which computers — like human beings —automatically pick up on patterns in their learning environment. Photo: Department of Computer Sciences/UW-Madison
Compared to the relentless growth of computer processing power and seemingly bottomless expansion of digital storage space, human expertise looks like a rare commodity.
But that’s not to say the digital and the cerebral are at odds. A growing group of University of Wisconsin–Madison researchers is working on ways to use computers to make better use of human brain power.
“We’re coming from psychology and educational psychology, from engineering and computer sciences, from biology and medicine,” says Tim Rogers, a University of Wisconsin–Madison psychology professor and principal investigator of LUCID, or Learning, Understanding, Cognition, Intelligence and Data Science. “That gives you an idea of the variety of spheres of human life that involve algorithms working in an independent sort of way with data provided by human users, and even deciding what to feed back to the humans.”
LUCID began in 2015 with a National Science Foundation grant to train graduate students in the cross-disciplinary skills necessary to work on problems with the help of machine learning, in which computers, like human beings, automatically pick up on patterns in their learning environment. But it was five years before that that Rogers and LUCID co-leader Rob Nowak, an electrical and computer engineering professor, tackled for the U.S. Air Force the problem of a flood of satellite and aerial photography washing over the few experienced and trusted human analysts assigned to interpret the pictures.
The process of examining satellite images is hard to automate, because often the experts don’t know what they’re looking for until they see it.
“In cases like this, the bottleneck is the human resource. It’s limited, expensive and slow, but very necessary,” says Nowak, who has designed a system, called NEXT, in which computers cooperate with people to quickly solve tasks beyond what can be achieved by computers alone.
Armed with a poor eye for what is useful, computers can make very fast — but not very accurate — decisions about which images include important stuff. Humans can do an excellent job of picking out those key features, but at a snail’s pace.
“The machine would use those human votes to improve its own search. And together they more efficiently find interesting things than either would on its own.”
Tim Rogers
What the machine learning researchers could do was mate the complementary skills. The computer used imperfect machine algorithms to rank order the images. Human analysts began reviewing the pictures the computer thought were most important, indicating the ones that contained objects of interest, like vehicles or people.
“The machine would use those human votes to improve its own search,” Rogers says. “And together they more efficiently find interesting things than either would on its own.”
That may be the most lucid description of the type of research LUCID tends to attract — studies that leverage machine learning to make better, quicker work of questions that involve hefty data loads and tricky comparisons of signal versus noise.
The program has four core areas of research: developing and applying the NEXT system, decoding brain imaging data, computer sciences Professor Bilge Mutlu’s work developing robots that do a better job interacting with people, and machine teaching — an effort led by computer sciences Professor Jerry Zhu that turns machine learning on its head to improve the way human students learn.
“Usually the idea with machine learning is that you have a lot of data, and you’re trying to figure out a good learning model to represent this data,” Rogers says. “In the machine teaching case, the idea is we have a model of the learner — it’s based on laboratory studies of learning in cognitive psychology — and we want to know which lessons will get the learner to pick up some concept quickly.”
Nowak’s lab has used NEXT to advance the work of psychologists like Rogers and UW–Madison Professor Paula Niedenthal, whose work on emotions involves the way people perceive facial expressions such as smiles.
NEXT can take on studies in which people rate things — from most trustworthy to least, for example — or compare one smile or object or word to another.
“NEXT allows us to go beyond what you could do conventionally in a completely randomized experimental design, but in a very controlled way. It accelerates the whole process, and allows us to move on to much larger problems.”
Rob Nowak
Building a large sample for that kind of study can be incredibly time-consuming, and a daunting data management task.
“The traditional approach to experiments like this is to bring a lot of undergrads into a room with a computer and sit them down to answer questions,” says Lalit Jain, a graduate student in Nowak’s lab.
Asking people to compare pairs of smiles from a set of 10 involves 45 questions for each person in the study. Increasing the number of smiles to 100 makes almost 5,000 unique pairs and a huge investment of survey time.
But the NEXT system, learning as it collects answers from study participants, can adjust to eliminate unnecessary questions. If the difference between two smiles is strong after a representative group of participants have made that decision, NEXT can decide not to bother the rest of its human panel with that comparison — and instead put them to work on untangling less clear results.
“NEXT allows us to go beyond what you could do conventionally in a completely randomized experimental design, but in a very controlled way,” Nowak says. “It accelerates the whole process, and allows us to move on to much larger problems.”
And, Jain points out, because NEXT can run studies online, researchers can use web-based services to recruit participants from cultures and demographics not readily at hand even on the campus of a large Midwestern research university.
Rebecca Willett — another professor of electrical and computer engineering, a fellow of the Wisconsin Institute for Discovery’s Optimization Group and one of LUCID’s core group of faculty — is working with Rogers and Nowak to use machine learning to sort out the useful bits of information in brain scans.
Functional MRI (fMRI) is used to record the flow of oxygen throughout the brain, and how that flow changes in response to external stimuli. Neuroscientists hope this data can be used to infer how the brain is encoding information, but significant data science challenges make this a difficult task.
“If you look over time at how much oxygen is in a part of the brain, that’s going to fluctuate a little bit no matter what external stimulus is present,” Willett says. “This ‘noise’ and other physiological factors can confound traditional decoding methods.”
LUCID students work in internships of sorts that take on real-world problems for nonacademic partners such as The New Yorker — where NEXT is helping the cartoon editor manage thousands of entries in the magazine’s weekly caption contest.
To make statistically sound conclusions, researchers often have to focus on small regions of the brain they assume are involved in the particular function they’re studying.
“The problem is, it’s not clear that assumption is true,” Willett says. “And if we make that assumption every time we analyze data, we risk biasing our fundamental understanding of how the brain works.”
Machine learning can be used to sidestep that risk.
“Our litmus test,” Willett says, “is whether we can examine fMRI data and determine what someone was doing or experiencing during the scan. The better our inferences, the more likely our model of how the brain is working is accurate, and the lower the risk that we excluded something important.”
The promise of machine learning has caught the attention of more than the Air Force, according to Nowak, who watched attendance at a major conference on the subject flip in recent years.
“In the past, 90 percent of people attending were like me, professors or students presenting papers. It was a very scientific meeting,” he says. “But this year, maybe half of the people were there not to present, but to hear about research and to recruit students for jobs.”
“This new website will help new students, faculty, and industry partners see the strength of machine learning research at UW.”
Rebecca Willett
LUCID students work in internships of sorts that take on real-world problems for nonacademic partners such as Technicolor, Microsoft Research and The New Yorker magazine — where NEXT is at work helping the cartoon editor manage thousands of entries in the magazine’s weekly caption contest.
“It’s a way to get our students more experience outside research and academic training, and also sort of on the employer side to make them aware of the skills our students will have when they come out of the program,” Rogers says.
The researchers are also working to expand their numbers, reaching out to other potential collaborators and students on campus — including the transdisciplinary Machine Learning @ UW–Madison group.
“Fundamental machine learning research is being conducted in departments of Computer Sciences, Electrical and Computer Engineering, Statistics, Mathematics and Biostatistics. Many more researchers, spread across the UW–Madison campus and colleges, are exploring the use of machine learning in novel science and engineering contexts,” says Willett. “This new website will help new students, faculty, and industry partners see the strength of machine learning research at UW.”
Tags: computer science, computers, learning, psychology